I’ve been following the noise since ChatGPT was launched a few months ago, and am struck by the way that it seems to have fired up some of the old tropes about the inevitability of technology that emerged much earlier in the long digital wave. I’ve also seen some excitable chat about how AI is, somehow, going to be the backbone of the next long technology surge. Both of these assertions seem unlikely to me.
Regular readers will know that I tend to go back to Carlota Perez’ model, or heuristic, of how long technology surges work when I’m thinking about technology, and I do this because it has been a pretty reliable model which has over 20 years acted as a decent and testable theory of change.
To summarise Perez in a single paragraph: there have been five technology surges since 1771, each lasting 50-60 years. Each surge involves an installation phase, funded by finance or investment capital; then there’s a crash; then there’s a deployment phase, funded by production capital. She doesn’t describe it like this, but market share at the time of the crash is typically somewhere between early adopter and early majority.
External costs
One of my deductions from using the Perez model over the last 20 years is that by the time it reaches the end of its S-curve, the external costs of the technologies are all too visible, and as a result regulators are all over it and critics get heard.
We’re seeing this with AI, or more exactly Large Learning Models (LLMs) at the moment. I’m going to pass here on the much-trumpeted letter that called for a ‘pause’ to AI development, partly because it seemed to me to be a badly thought through piece of self-interested virtue signalling.
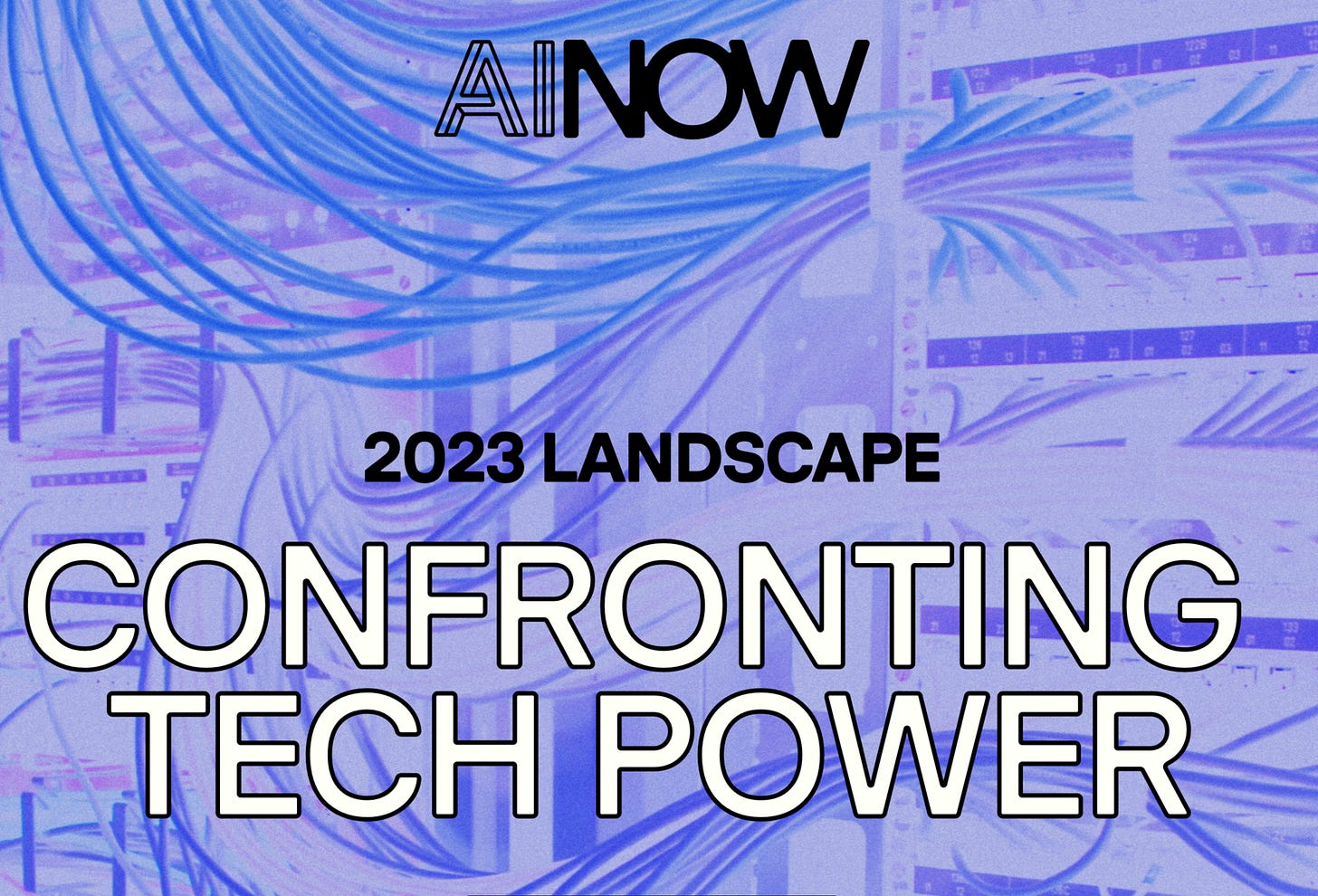
The more recent paper from the AI Now Institute, covered in Vox, seems to be closer to the mark, partly because it was written by a couple of former Federal Trade Commission regulators who understand how regulation works. (My thanks to my former colleague Andre Furstenburg for alerting me to it.)
Part of their argument is about market power:
To build state-of-the-art AI systems, you need resources — a gargantuan trove of data, a huge amount of computing power — and only a few companies currently have those resources. These companies amass millions that they use to lobby government; they also become “too big to fail,” with even governments growing dependent on them for services… “A handful of private actors have accrued power and resources that rival nation-states while developing and evangelizing artificial intelligence as critical social infrastructure,” the report notes.
Burdens of proof
Well, you can’t have it both ways, they suggest. If it is a critical social infrastructure, then private developers need to be able to demonstrate that there are not harms built in to their development:
(T)he report’s top recommendation is to create policies that place the burden on the companies themselves to demonstrate that they’re not doing harm. Just as a drugmaker has to prove to the FDA (Food and Drug Administration) that a new medication is safe enough to go to market, tech companies should have to prove that their AI systems are safe before they’re released.
This would, for example, be one way to deal with the current set of issues around bias in AI, which, famously, Google researchers were fired for pointing out.
Saturated markets
And the comparison with the FDA is an interesting one, since—as we can see from the current waves of redundancies—when technology surge markets get saturated (typically somewhere around the beginning of the fourth quarter of the S-curve), they stop gaining ‘free’ growth from new customers and new applications, and become more like other companies which have to worry about margins and rates of return and optimising their product portfolios.
Part of the point here is that issues like market power are being talked about quite noisily; part of the point is that mainstream coverage, such as that seen in Vox, is now as likely to be critical as positive. Again, for obvious reasons, this happens at the end of the surge, not at the beginning. (The AI Now Institute report is here.)
This is one of the reasons why AI is unlikely to drive a new generation of rapid technical innovation—there simply isn’t enough money in it. Again, Perez doesn’t quite put it like this, but at the start of each of her five surges there is a significant innovation, sometimes only seen retrospectively, that creates a new form of abundance through radical cost reduction, which then opens up a transformative new market. It’s this that attracts the finance capital in. (Think: Crompton’s Spinning Jenny, or Ford’s assembly line, or the invention of the microprocessor.)
The money in AI
The money in AI? Well, on this topic, Byrne Hobart’s finance newsletter The Diff had an interesting piece in its outside-the-paywall coverage back in January.
Was the market in AI applications, he asked, more like the steel industry, or more like a software application like Visual Basic? He’s not denying that AI applications are getting simpler and cheaper, and that therefore they will create new use cases. In fact that is his starting point. But:
What if they’re the next steel industry? Steel is a useful and ubiquitous product; this post opening an upcoming series on the steel industry notes that “Nearly every product of industrial civilization relies on steel, either as a component or as part of the equipment used to produce it.”—but that doesn’t make it a great business.
Steel is a capital-intensive, cyclical business, with high fixed costs. Workers have the ability to negotiate good wages in the upcycle. Capacity is always higher than demand, because governments see it as a strategic industry. And because of this last factor, governments will also have a view on who AI businesses can sell to or buy from (see also Huawei and TikTok). And remaining competitive involves building ever larger LLMs, drawing on the same sets of data as your competitors:
So that’s the pessimistic view for investors: AI will be as important and ubiquitous as a product, like steel, but AI companies will be relatively minor players in the economy they prop up.
The optimistic version
And then there’s the optimistic version: AI as an analogue for Visual Basic. If this seems like an odd choice:
This case is compelling because large language models are a nice natural language glue between a) software products that don’t have good APIs, or b) mixed software-and-human processes that are tricky to fully automate…. The world’s many companies running some form of legacy software, with idiosyncratic levels of automation and organizations partly built around where they choose to have humans in the loop, will benefit from AI tools that connect these systems together. And what most of these businesses almost certainly have in common is that they’re almost certainly running Microsoft software.
This makes AI products a high value niche, where software is valuable and humans are cheaper. And it is possible to see a market here which is more attractive than trying to sell advertising off the back of some kind of enhanced search product. It’s just not the kind of market that drives the next technology surge. [1]
Productivity tools
More recently, the technology blogger Dave Karpf seems to have come to a similar conclusion: that the value in our current set of AI tools is likely to be as a suite of productivity tools:
Where I think this will be most transformative is in online productive tools. We are probably approaching a future where Microsoft unveils a legitimately awesome next-generation Clippy.

‘Clippy’ was a digital assistant created by Microsoft in the late 1990s to help people use their computers, which developed a cult following even after it was killed off. But Karpf is sceptical about whether that generates enough income to sustain the burn rate of some of the leading AI developers. There’s quite a big mismatch:
OpenAI burned through $540 million developing ChatGPT last year. Sam Altman has suggested they’ll need $100 billion to develop the AI of his dreams. There is not $100 billion+ of revenues to be found in Clippy-but-awesome…
Over time, the trajectory of every new technology bends toward money. There are reasons to be excited about the ways this new technology might simplify our lives. It’s going to make satisficing so much easier, and that is often just what we need. But we should also watch the emerging revenue models closely.
Before someone misunderstands this article, I do believe that AI will have significant social effects. Again, Perez doesn’t write about this, but there’s a period after the end of the surge when there is significant socio-economic innovation around the now mature technology: think of the development of logistics and just-in-time business models in the 1970s. I think that’s the right analogy for AI: the aftershock of the digital surge, not the harbinger of the next technology surge.
——
A version of this article is also published on my Just Two Things Newsletter.
Notes
[1] I’m on the record as saying that the next Perez surge is likely to be driven by some form of synthetic biology or some kind of transformative materials technology.
I think that you are correct on this. I can’t see the revenue streams that justify the investments yet. The huge valuations of incumbents require next stage innovations to sustain the growth patterns. This feels more like the end of an S-Curve not the start of a new. As an example, think of the peak of the mainframe or minicomputer waves in the 70s and 80s.Next wave minis were overtaken my the PC, especially when networked. I sat in on a “Mainframe 2000” presentation in the 1980s in the US. It was well sold and argued, but never happened.